Papers Explained 64: Mistral

Mistral 7B is an LLM engineered for superior performance and efficiency. It leverages grouped-query attention (GQA) for faster inference, coupled with sliding window attention (SWA) to effectively handle sequences of arbitrary length with a reduced inference cost.
Mistral 7B outperforms the best open 13B model (Llama 2) across all evaluated benchmarks, and the best released 34B model (Llama 1) in reasoning, mathematics, and code generation.
Mistral 7B — Instruct, model fine-tuned to follow instructions on instruction datasets publicly available on the Hugging Face repository, surpasses Llama 2 13B — chat model both on human and automated benchmarks.
Architecture
Mistral 7B is based on a transformer architecture.

Compared to Llama, it introduces a few changes:
Sliding Window Attention

Sliding Window Attention leverages the layers of a transformer model to extend its attention beyond a fixed window size, denoted as W. In SWA, the hidden state at position i in layer k can attend to hidden states from the preceding layer within the range of positions i — W to i, allowing access to tokens at a distance of up to W * k tokens. By employing a window size of W = 4096, SWA theoretically achieves an attention span of approximately 131K tokens. In practice with a sequence length of 16K and W = 4096, SWA modifications in FlashAttention and xFormers result in a 2x speed enhancement compared to vanilla attention methods.
Rolling Buffer Cache

A Rolling Buffer Cache, employs a fixed attention span to limit cache size. The cache is of fixed size W, and it stores keys and values for timestep i at position i mod W in the cache. When i exceeds W, earlier values are overwritten, halting cache size growth. For instance, with W = 3, on a 32k-token sequence, cache memory usage is reduced by 8x without compromising model quality.
Pre-fill and chunking

In sequence generation, tokens are predicted sequentially based on prior context. To optimize efficiency, a (k, v) cache is pre-filled with the known prompt. If the prompt is very long, it is chunked into smaller segments using a chosen window size. Each chunk is used to pre-fill the cache. This approach involves computing attention both within the cache and over the current chunk, Thus aiding in more effective sequence generation.
Results
Mistral is evaluated against the following benchmarks:
- Commonsense Reasoning (0-shot): Hellaswag, Winogrande, PIQA, SIQA, OpenbookQA, ARC-Easy, ARC-Challenge, CommonsenseQA
- World Knowledge (5-shot): NaturalQuestions, TriviaQA
- Reading Comprehension (0-shot): BoolQ, QuAC
- Math: GSM8K (8-shot) with maj@8 and MATH (4-shot) with maj@4
- Code: Humaneval (0-shot) and MBPP (3-shot)
- Popular aggregated results: MMLU (5-shot), BBH (3-shot), and AGI Eval (3–5-shot, English multiple-choice questions only)
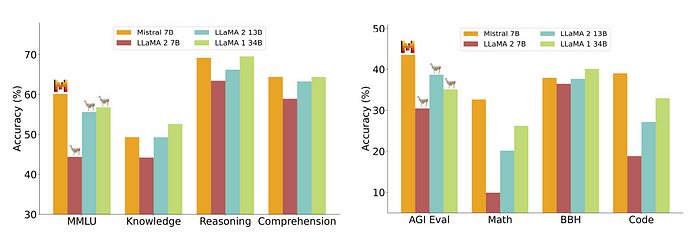

- Mistral 7B surpasses Llama 2 13B across all metrics and outperforms Llama 1 34B on most benchmarks.
- In particular, Mistral 7B displays superior performance in code, mathematics, and reasoning benchmarks.
Instruction Following
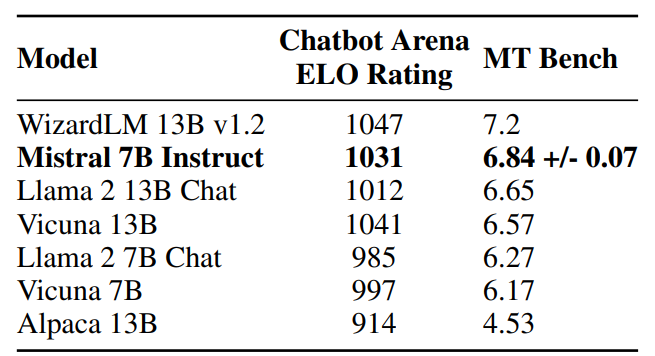
- Mistral 7B — Instruct, outperforms all 7B models on MT-Bench, and is comparable to 13B — Chat models.
- In an independent human evaluation, conducted on https://llmboxing.com/leaderboard. The outputs generated by Mistral 7B were preferred 5020 times, compared to 4143 times for Llama 2 13B.
Mistral 7B-v0.2
Mistral-7B-v0.2 has the following changes compared to Mistral-7B-v0.1
- 32k context window (vs 8k context in v0.1)
- Rope-theta = 1e6
- No Sliding-Window Attention
Mistral 7B-v0.3
Mistral-7B-v0.3 has the following changes compared to Mistral-7B-v0.2
- Extended vocabulary to 32768
- Supports v3 Tokenizer
- Supports function calling
Codestral 22B
Codestral is a 22B open-weight code model, specifically designed for code generation tasks, which is an open-weight generative AI model. It is trained on a diverse dataset of over 80 programming languages, including popular ones like Python, Java, C, C++, JavaScript, and Bash, as well as more specific ones like Swift and Fortran. This broad language base enables Codestral to assist developers in various coding environments and projects.
Codestral can save developers time and effort by completing coding functions, writing tests, and filling in partial code using a fill-in-the-middle mechanism. Interacting with Codestral can help developers improve their coding skills and reduce the risk of errors and bugs.
Codestral is licensed under the Mistral AI Non-Production License, allowing it to be used for research and testing purposes.
Setting the Bar for Code Generation Performance

As a 22B model, Codestral sets a new standard on the performance/latency space for code generation compared to previous models used for coding.
With its larger context window of 32k (compared to 4k, 8k or 16k for competitors), Codestral outperforms all other models in RepoBench, a long-range eval for code generation.

Additionally, Codestral’s performance is evaluated in multiple HumanEval pass@1 across six different languages in addition to Python: C++, bash, Java, PHP, Typescript, and C#, and calculated the average of these evaluations.

Codestral’s Fill-in-the-middle performance was assessed using HumanEval pass@1 in Python, JavaScript, and Java and compared to DeepSeek Coder 33B, whose fill-in-the-middle capacity is immediately usable.
Mathstral
Mathstral is a 7B model designed for math reasoning and scientific discovery based on Mistral 7B specializing in STEM subjects. It achieves state-of-the-art reasoning capacities in its size category across various industry-standard benchmarks. The model has a 32k context window.
In particular, it achieves 56.6% on MATH and 63.47% on MMLU.


Mathstral can achieve significantly better results with more inference-time computation: Mathstral 7B scores 68.37% on MATH with majority voting and 74.59% with a strong reward model among 64 candidates.
Mistral Nemo
Mistral NeMo is a 12B language model built in collaboration with NVIDIA. It features a large context window of up to 128k tokens and state-of-the-art reasoning, world knowledge, and coding accuracy.
The model is available as pre-trained base and instruction-tuned checkpoints. The model was trained with quantization awareness, enabling FP8 inference without any performance loss.
The models are available at HuggingFace: Base Model and Instruct Model.
Tekken Tokenizer

Mistral NeMo uses a new tokenizer called Tekken, which was trained on over 100 languages. Tekken compresses natural language text and source code more efficiently than the SentencePiece tokenizer used in previous Mistral models.
Evaluation
Benchmark Results

Multilingual Model

Mistral NeMo is designed for global, multilingual applications. It is trained on function calling and has a large context window. The model performs well in multiple languages, including English, French, German, Spanish, Italian, Portuguese, Chinese, Japanese, Korean, Arabic, and Hindi.
Instruction Fine-tuning

Mistral NeMO underwent advanced fine-tuning and alignment to improve its ability to follow precise instructions, reason, handle multi-turn conversations, and generate code.
Mistral Large 2
Mistral Large 2 is a 123B model, offering significant improvements in code generation, mathematics, and reasoning capabilities compared to its predecessor. It also provides advanced function calling capabilities. It has a 128k context window. It Support for dozens of languages (including French, German, Spanish, Italian, Portuguese, Arabic, Hindi, Russian, Chinese, Japanese, and Korean) and over 80 coding languages (such as Python, Java, C, C++, JavaScript, and Bash).
The model is available at HuggingFace.
Evaluation
General performance
- Achieves an accuracy of 84.0% on MMLU, setting a new point on the performance/cost Pareto front of open models
Code & Reasoning

- Vastly outperforms previous Mistral Large model and performs on par with leading models such as GPT-4o, Claude 3 Opus, and Llama 3 405B
- Minimized the tendency to “hallucinate” or generate plausible but factually incorrect information through fine-tuning
- Trained to be more cautious and discerning in its responses, ensuring reliable and accurate outputs
- Improved performance on popular mathematical benchmarks, demonstrating enhanced reasoning and problem-solving skills
Instruction following & Alignment

- Drastically improved instruction-following and conversational capabilities
- Better at following precise instructions and handling long multi-turn conversations
- Average length of generations remains succinct and to the point whenever possible
Language diversity

- Excels in English, French, German, Spanish, Italian, Portuguese, Dutch, Russian, Chinese, Japanese, Korean, Arabic, and Hindi
- Performs well on the multilingual MMLU benchmark compared to previous models
Tool Use & Function Calling
- Trained to proficiently execute both parallel and sequential function calls

Mistral Small
Mistral Small v24.09 is an upgrade of Mistral Small v24.02.
With 22B parameters, Mistral Small v24.09 offers a convenient mid-point between Mistral NeMo 12B and Mistral Large 2. It delivers significant improvements in human alignment, reasoning capabilities, and code over the previous model.
It has context length of 128K, and supports function calling.
The model is available at HuggingFace.

Ministral
Ministral are 3B and 8B are models for on-device computing and at-the-edge use cases. Both models support up to 128k context length (currently 32k on vLLM). Ministral 8B has a special interleaved sliding-window attention pattern for faster and memory-efficient inference and is available on HuggingFace.

Pre-trained Models

Fine Tuned Models

Mistral Small 3
Mistral Small 3 is a 24-billion parameter, latency-optimized language model released under the Apache 2.0 license. Designed for the “80%” of generative AI tasks requiring robust language and instruction following with low latency, it’s optimized for local deployment.
- Performance: Achieves over 81% accuracy on MMLU. Competitive with larger models like Llama 3.3 70B and Qwen 32B, and outperforms proprietary models like GPT4o-mini in speed and some benchmarks.
- Multilingual: Supports numerous languages, including English, French, German, Spanish, Italian, Chinese, Japanese, Korean, Portuguese, Dutch, and Polish.
- Context Window: 32k

- Mistral Small 3 is competitive with larger models such as Llama 3.3 70B or Qwen 32B, and is an excellent open replacement for opaque proprietary models like GPT4o-mini.
- Mistral Small 3 is on par with Llama 3.3 70B instruct, while being more than 3x faster on the same hardware.



- Mistral Small 3 offers the best performance for its size class and rivals with models three times larger such as Llama 3.3 70B.
Mistral Saba
Mistral Saba is a specialized regional language model designed to address the growing need for AI that understands cultural nuances and regional parlance. It’s a 24B parameter model specifically trained on curated datasets from the Middle East and South Asia, with particular strength in South Indian languages like Tamil. Despite being a 24B parameter model, it outperforms models over five times its size in terms of accuracy and relevance, while also offering faster response times (over 150 tokens per second) and lower costs.
Mistral Small 3.1
Mistral Small 3.1 is a new open-source language model that boasts best-in-class performance for its size. It builds upon its predecessor, Mistral Small 3, with significant improvements in several key areas:
- Improved Text Performance: General improvements in text-based tasks. Specific benchmarks and metrics detailing the improvements are not explicitly provided.
- Multimodal Understanding: Enhanced ability to process and understand information from multiple modalities (e.g., text and images). Performance metrics are visualized in provided charts comparing Mistral Small 3.1 to other models on benchmarks like MM-MT-Bench, ChartQA, DocVQA, and AI2D.
- Expanded Context Window: Increased context window of up to 128k tokens, enabling the model to handle significantly longer input sequences compared to its predecessor. Performance is visualized in a chart comparing it to other models on LongBench v2 and RULER benchmarks.
- Faster Inference Speed: Delivers inference speeds of 150 tokens per second.



Hungry for more insights?
Don’t miss out on exploring other fascinating threads in this series. Simply click here and uncover the state-of-the-art research!
Do Subscribe for weekly updates!!